In the ever-evolving world of legal technology, the emergence of large language models may revolutionise the way legal professionals work. These advanced models, such as GPT-3.5, have paved the way for a new generation of legal software applications. However, it is essential to understand the key differences between legal software based on large language models and those built on proprietary models to make informed decisions. This article aims to provide an overview of these differences and highlight the pros and cons of both approaches.
Understanding Large Language Models: Large language models are trained on vast amounts of data, allowing them to generate human-like text and comprehend complex language patterns. They can assist legal professionals in various tasks, including contract analysis, legal research, document generation, and more. Legal software based on these models utilises their inherent capabilities to enhance efficiency and accuracy.
Proprietary Models: Proprietary models, developed by legal software providers themselves, are tailored specifically for legal tasks. These models are built using proprietary algorithms and trained on domain-specific legal data. They offer a more focused and specialised approach to legal software development.
Differences between Legal Software based on Large Language Models and In-House Models are three-fold:
a. Training Data:
Legal software based on large language models benefits from being trained on diverse datasets, which include a wide variety of legal texts. This extensive training helps these models understand legal language better, allowing them to offer suggestions that are relevant to the context. On the other hand, proprietary models are trained on specific legal datasets, making them more skilled at specialised legal tasks. However, this specialisation might limit their ability to handle a broader range of legal tasks.
b. Generalisation vs. Specialisation:
Legal software based on large language models is excellent at handling different legal tasks because it can recognise patterns and understand concepts across various domains. It can adapt to different legal situations with minimal fine-tuning, which is known as "zero-shot" learning. In contrast, proprietary models provide specialised solutions designed for specific legal domains or specific uses. They may perform better than large language models in narrow areas but require more development and maintenance efforts.
c. Legal Expertise:
Legal software based on large language models uses the extensive knowledge acquired from their training data, which includes legal precedents, court decisions, and legal literature. This allows them to provide insights and recommendations based on a wide range of legal information. On the other hand, proprietary models rely more heavily on the expertise and domain knowledge of the software development team, even though they are trained on legal data.
Pros and Cons:
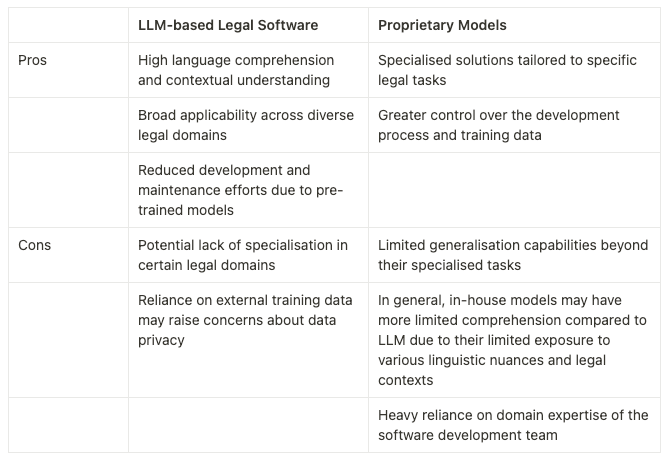
Conclusion:
With their broad understanding of legal language, adaptability across domains, and ability to handle diverse legal tasks, LLMs offer significant advantages. While proprietary models may excel in specialised legal domains, they often require extensive development and maintenance efforts, limiting their broader applicability. In contrast, LLMs provide a more versatile and efficient approach, leveraging their extensive training on diverse datasets to deliver superior results. Although data privacy and bias concerns associated with LLMs should be addressed, these issues can be mitigated through proper safeguards and ethical practices. Considering the transformative impact of LLMs, harnessing their power while ensuring responsible use is key.
Comments